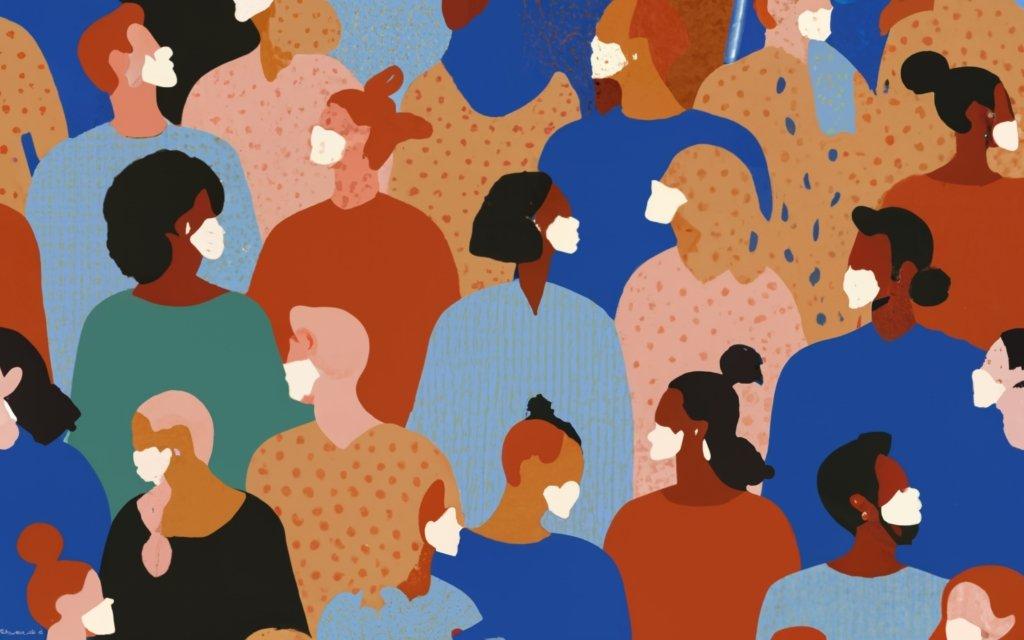
The line between reality and artificial fabrication is increasingly blurred by the onset of AI 'deepfakes'. Deepfakes are highly realistic digital creations made by combining "deep learning" with fake imagery. Deepfake detection is crucial, because it poses a significant threat to the authenticity of information, potentially leading to misinformation and manipulation on an unprecedented scale.
The rapid evolution of this technology means that deepfake detection methods must constantly evolve to identify and counter new techniques. The development of effective deepfake detection tools is crucial for maintaining truth and trust in the digital world.
The Rising Challenge of Deepfakes
Deepfakes are made using deep learning, a type of artificial intelligence. They specifically use techniques like Generative Adversarial Networks (GANs). In this process, two neural networks, 'generator' and 'discriminator' work in tandem. The realism of deepfakes has been increasing due to advancements in AI.
Undetected deepfakes pose significant risks, including personal character damage, political manipulation and loss of trust in media, making deepfake detection increasingly vital.
Basic Principles of Deepfake Detection
Deepfake detection hinges on identifying discrepancies that are typically absent in authentic media. These include:-
- Subtle inconsistencies in facial expressions.
- Unnatural blinking or lip movements.
- Irregularities in skin texture.
- Disalignment of lighting and shadows with the environment.
- Mismatches in audio-visual synchronisation.
Digital forensics also plays a crucial role in deepfake detection. It involves the examination and analysis of digital data to uncover anomalies and signs of manipulation.
Machine Learning and AI in Deepfake Detection
Machine learning (ML) plays a pivotal role in deepfake detection. ML algorithms are trained on a vast dataset comprising both authentic and deepfake media. This training involves exposing the algorithm to numerous examples, allowing it to learn and identify the subtle differences between real and manipulated content.
AI automates the process of deepfake detection and continuously improves it. Once trained, AI systems can quickly analyse new videos or images, making real-time deepfake detection feasible. As these AI algorithms are exposed to newer versions of deepfakes, they can adjust their deepfake detection mechanisms to stay ahead of the evolving techniques used by deepfake creators.
Specific Deepfake Detection Tools and Techniques
Facial Recognition and Analysis
Advanced facial recognition technologies are employed to detect anomalies in facial features. Despite its effectiveness, facial analysis has limitations. High-quality deepfakes can sometimes bypass these deepfake detection methods.
Analysing Digital Footprints
- Metadata analysis involves examining the digital information embedded in media files.
- Digital artefacts are inconsistencies or flaws left behind during the deepfake creation process.
Various software tools are available for metadata and artefact analysis. These tools scrutinise the file’s data to reveal signs of tampering or inconsistencies that suggest manipulation.
Behavioural and Movement Analysis
This involves analysing the subject’s movements and expressions for any signs of artificiality such as irregular head movements or facial expressions that don’t sync with the spoken words.
Audio Analysis
Audio analysis is critical in deepfake detection, focusing on identifying mismatches in voice timbre, speech patterns, and lip-sync errors.
Consistency and Context Checks
This involves checking & verifying the content, the background, the context, and other elements in the video or image for inconsistencies.
Emerging Technologies in Deepfake Detection
Deepfake detection is rapidly evolving, especially with these latest advancements:
- Use of blockchain for content verification.
- Convolutional Neural Networks (CNNs)
- Recurrent Neural Networks (RNNs).
- Integration of AI with real-time detection capabilities.
- Use of quantum computing in deepfake detection.
The implications of these advancements are far-reaching. They could greatly enhance the security of information in fields such as journalism, social media and national security.
Challenges in Deepfake Detection
The development of deepfake technology and its detection is often described as an arms race, with each side continuously evolving to outdo the other. As deepfake algorithms become more sophisticated, they produce increasingly realistic and harder-to-detect fakes. In response, deepfake detection technologies are also advancing, employing more complex algorithms and diverse techniques to identify fakes.
Current deepfake detection technologies face challenges like false positives and negatives, difficulty in analysing low-quality videos and the need for extensive, diverse training datasets.
The Role of Policy and Education
Legal and regulatory measures are critical in deepfakes detection, as they establish clear definitions and penalties for malicious creation and distribution. Increasing public awareness and education about deepfakes is vital to empower individuals to critically assess digital content. This approach fosters a more informed public, capable of discerning authenticity in digital media and reducing the bad impact of deepfakes.
Conclusion
Deepfake detection is critical in maintaining the integrity of digital media and safeguarding information dissemination. Deepfake detection tools and techniques, ranging from advanced ML algorithms to digital forensics, facial recognition and audio analysis, represent significant strides in identifying and countering these sophisticated digital forgeries. These technologies, alongside emerging methods like blockchain verification and hybrid detection systems, form a robust arsenal in the fight against deepfake threats.
The rapid advancement of deepfake technology necessitates constant vigilance and ongoing research. As creators of deepfakes continue to refine their methods, deepfake detection tools must evolve correspondingly to stay ahead.